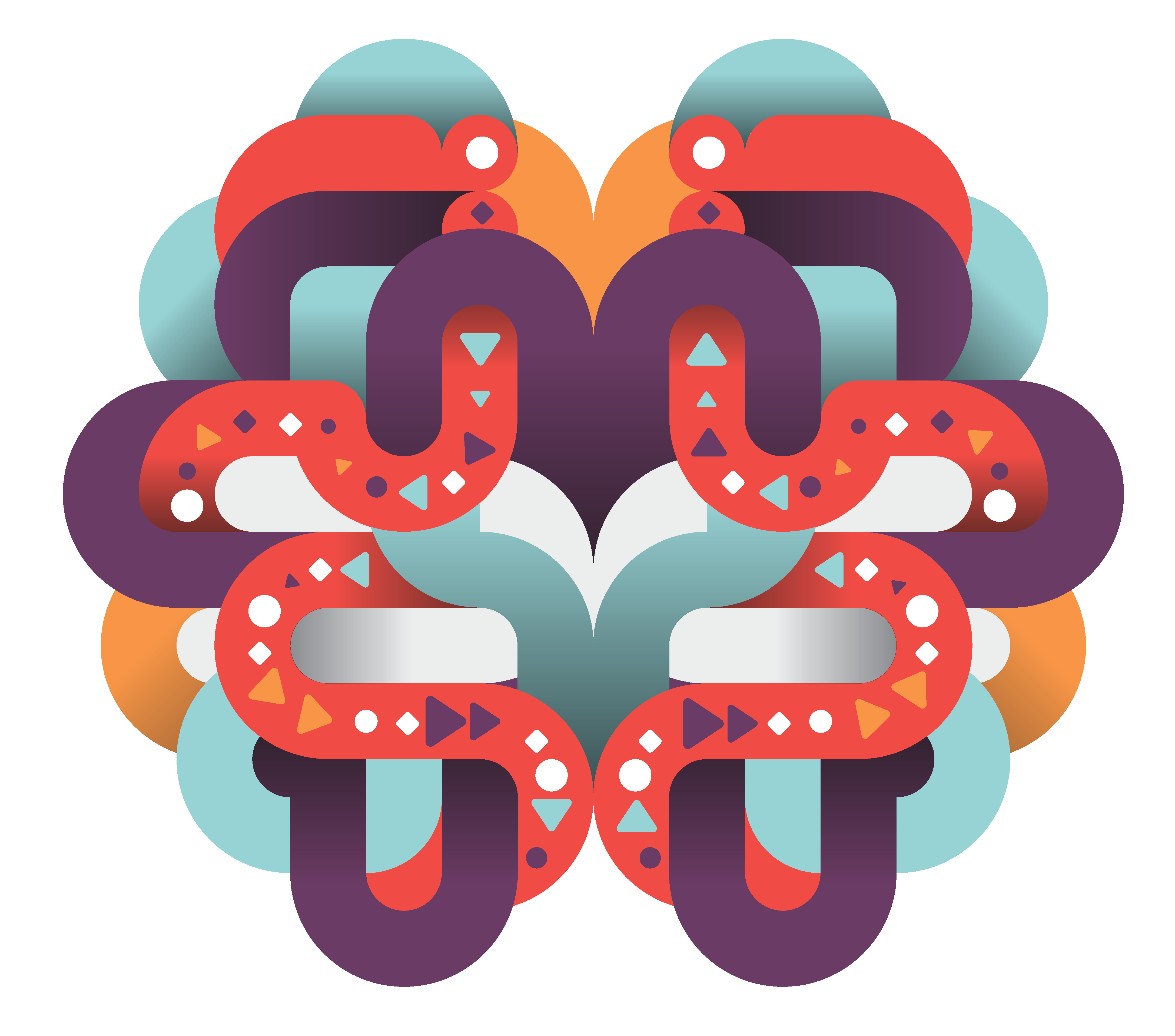
Mitigating sensory overload with
closed-loop stimulation
The ability of the brain to generate predictions about what we see, hear, and so on, automatically, and unconsciously on a second-by-second basis is key to perception. Predicted inputs are filtered out, while unexpected inputs, known as “prediction errors,” are processed and used to update the brain’s model. Dysfunction in this process of “predictive coding” may explain some features of autism, such as abnormal prediction signals that lead to too many false prediction errors and an oversensitivity to sensory stimulation, making it difficult to process social cues, learn, and communicate 1-5.
There is mounting evidence that neural oscillations (brain waves) play a central role 6,7. According to the Predictive Routing model, alpha/beta waves feedback predictions. They regulate the gamma feedforwarding prediction errors. Sensory overload results from their imbalance. The feedforward gamma waves overwhelm the feedback alpha/beta waves. Autistics show alterations in both alpha/beta and gamma bands and alterations in processing of prediction errors 8,9.
This project will test this hypothesis via direct, cause-and-effect, manipulations of brain waves in macaque monkeys and shank 3 marmosets (a genetic model of a human disorder – Phelen McDermid Syndrome – that is strongly associated with autism). We developed new ultra-fast closed-loop electrical stimulation technology that can increase and decrease the alpha/beta prediction waves. The model will also be tested in EEG recordings from ASD children.
Project 1 will test the hypothesis that turning down alpha/beta should reduce prediction signals, producing the altered predictive coding seen in autistics (Figure 1). Turning up alpha/beta should mitigate this by improving predictive coding. Project 2 will test for the rhythmic imbalance in shank 3 marmosets, their relation to oddball/predictive processing and whether normal processing can be restored via closed-loop stimulation. Project 3 will test for the rhythmic imbalance and their relationship to oddball/predictive processing in ASD and PMS children. Our ultimate goal is non-invasive closed-loop stimulation for therapeutic use in humans.
Project 1: To use closed-loop manipulation of alpha/beta rhythms to test hypotheses about the functional circuitry of predictive coding (PI: Earl Miller). In macaque monkeys, we will determine if the effects of closed-loop manipulation of alpha/beta can replicate and mitigate deficits in predictive coding. Our main hypothesis is that decreasing prefrontal cortex alpha/beta via anti-phase electrical stimulation should reduce predictions, simulating altered prediction error processing seen in autism. By contrast, restoring alpha/beta back to full strength by phase-matched stimulation should have the opposite effect. It should strengthen predictions and restore the normal feeding forward of bottom-up sensory inputs. Further, the effects should be stimulus/pathway-specific. The alpha/beta effects should be larger at cortical sites where neurons prefer predicted stimuli and the gamma/spikes violation signals should be larger at sites that respond to a prediction-error stimulus.
Project 2: To assess rhythmic imbalance in shank 3 marmosets and restore balance using closed-loop stimulation (PI: Robert Desimone). We will compare shank 3 marmosets to wildtype marmosets to determine if they have alpha/beta rhythm imbalance. We will test the hypothesis that they show the altered predictive coding dynamics that characterize the macaque monkey cortex when we decrease alpha/beta. We will then determine if we can restore normal balance by manipulating their alpha/beta rhythms.
Project 3: To conduct parallel studies in children with autism (PI: Charles Nelson). We hypothesize that children with autism will show lower gamma power during rest and task than TD children; that autistic children will show a reduced amplitude P300 to the oddball/unpredicted stimuli; that the degree of functional impairment (greatest among those with PMS, followed by autism) will be inversely associated with beta and gamma activity (i.e., greater impairment will be associated with lower amplitude beta and gamma activity); and that source modeling will reveal more diffuse and less interconnected networks among children with autism compared to TD children.
References
1. Gonzalez-Gadea ML, Chennu S, Bekinschtein TA, Rattazzi A, Beraudi A, Tripicchio P, Moyano B, Soffita Y, Steinberg L, Adolfi F, Sigman M, Marino J, Manes F, Ibanez A. Predictive coding in autism spectrum disorder and attention deficit hyperactivity disorder. J Neurophysiol 2015;114:2625–36. https://doi.org/10.1152/jn.00543.2015.
2. Sinha P, Kjelgaard MM, Gandhi TK, Tsourides K, Cardinaux AL, Pantazis D, Diamond SP, Held RM. Autism as a disorder of prediction. PNAS 2014;111:15220–5. https://doi.org/10.1073/pnas.1416797111.
3. Cannon J, O’Brien AM, Bungert L, Sinha P. Prediction in Autism Spectrum Disorder: A Systematic Review of Empirical Evidence. Autism Research 2021;14:604–30. https://doi.org/10.1002/aur.2482.
4. Van de Cruys S, Evers K, Van der Hallen R, Van Eylen L, Boets B, de-Wit L, Wagemans J. Precise minds in uncertain worlds: Predictive coding in autism. Psychological Review 2014;121:649–75. https://doi.org/10.1037/a0037665.
5. Lawson RP, Rees G, Friston KJ. An aberrant precision account of autism. Front Hum Neurosci 2014;8. https://doi.org/10.3389/fnhum.2014.00302.
6. Bastos AM, Lundqvist M, Waite AS, Kopell N, Miller EK. Layer and rhythm specificity for predictive routing. PNAS 2020;117:31459–69. https://doi.org/10.1073/pnas.2014868117.
7. Bastos AM, Usrey WM, Adams RA, Mangun GR, Fries P, Friston KJ. Canonical microcircuits for predictive coding. Neuron 2012;76:695–711. https://doi.org/10.1016/j.neuron.2012.10.038.
8. O’Reilly C, Lewis JD, Elsabbagh M. Is functional brain connectivity atypical in autism? A systematic review of EEG and MEG studies. PLOS ONE 2017;12:e0175870. https://doi.org/10.1371/journal.pone.0175870.
9. Seymour RA, Rippon G, Gooding-Williams G, Schoffelen JM, Kessler K. Dysregulated oscillatory connectivity in the visual system in autism spectrum disorder. Brain 2019;142:3294–305. https://doi.org/10.1093/brain/awz214.